Some of the more unhinged writing on superintelligence pictures AI doing things that seem like magic. Crossing air gaps to escape its data center. Building nanomachines from simple components. Plowing through physical bottlenecks to revolutionize the economy in months.
More sober thinkers point out that these things might be physically impossible. You can’t do physically impossible things, even if you’re very smart.
No, say the speculators, you don’t understand. Everything is physically impossible when you’re 800 IQ points too dumb to figure it out. A chimp might feel secure that humans couldn’t reach him if he climbed a tree; he could never predict arrows, ladders, chainsaws, or helicopters. What superintelligent strategies lie as far outside our solution set as “use a helicopter” is outside a chimp’s?
Eh, say the sober people. Maybe chimp → human was a one-time gain. Humans aren’t infinitely intelligent. But we might have infinite imagination. We can’t build starships, but we can tell stories about them. If someone much smarter than us built a starship, it wouldn’t be an impossible, magical thing we could never predict. It would just be the sort of thing we’d expect someone much smarter than us to do. Maybe there’s nothing left in the helicopters-to-chimps bin - just a lot of starships that might or might not get built.
The first time I felt like I was getting real evidence on this question - the first time I viscerally felt myself in the chimp’s world, staring at the helicopter - was last week, watching OpenAI’s o3 play GeoGuessr.
GeoGuessr is a game where you have to guess where a random Google Street View picture comes from. For example, here’s a scene from normal human GeoGuessr:
The store sign says “ADULTOS”, which sounds Spanish, and there’s a Spanish-looking church on the left. But the trees look too temperate to be Latin America, so I guessed Spain. Too bad - it was Argentina. Such are the vagaries of playing GeoGuessr as a mere human.
Last week, Kelsey Piper claimed that o3 - OpenAI’s latest ChatGPT model - could achieve seemingly impossible feats in GeoGuessr. She gave it this picture:
…and with no further questions, it determined the exact location (Marina State Beach, Monterey, CA).
How? She linked a transcript where o3 tried to explain its reasoning, but the explanation isn’t very good. It said things like:
Tan sand, medium surf, sparse foredune, U.S.-style kite motif, frequent overcast in winter … Sand hue and grain size match many California state-park beaches. California’s winter marine layer often produces exactly this thick, even gray sky.
Commenters suggested that it was lying. Maybe there was hidden metadata in the image, or o3 remembered where Kelsey lived from previous conversations, or it traced her IP, or it cheated some other way.
I decided to test the limits of this phenomenon. Kelsey kindly shared her monster of a prompt, which she says significantly improves performance:
You are playing a one-round game of GeoGuessr. Your task: from a single still image, infer the most likely real-world location. Note that unlike in the GeoGuessr game, there is no guarantee that these images are taken somewhere Google's Streetview car can reach: they are user submissions to test your image-finding savvy. Private land, someone's backyard, or an offroad adventure are all real possibilities (though many images are findable on streetview). Be aware of your own strengths and weaknesses: following this protocol, you usually nail the continent and country. You more often struggle with exact location within a region, and tend to prematurely narrow on one possibility while discarding other neighborhoods in the same region with the same features. Sometimes, for example, you'll compare a 'Buffalo New York' guess to London, disconfirm London, and stick with Buffalo when it was elsewhere in New England - instead of beginning your exploration again in the Buffalo region, looking for cues about where precisely to land. You tend to imagine you checked satellite imagery and got confirmation, while not actually accessing any satellite imagery. Do not reason from the user's IP address. none of these are of the user's hometown. **Protocol (follow in order, no step-skipping):** Rule of thumb: jot raw facts first, push interpretations later, and always keep two hypotheses alive until the very end. 0 . Set-up & Ethics No metadata peeking. Work only from pixels (and permissible public-web searches). Flag it if you accidentally use location hints from EXIF, user IP, etc. Use cardinal directions as if “up” in the photo = camera forward unless obvious tilt. 1 . Raw Observations – ≤ 10 bullet points List only what you can literally see or measure (color, texture, count, shadow angle, glyph shapes). No adjectives that embed interpretation. Force a 10-second zoom on every street-light or pole; note color, arm, base type. Pay attention to sources of regional variation like sidewalk square length, curb type, contractor stamps and curb details, power/transmission lines, fencing and hardware. Don't just note the single place where those occur most, list every place where you might see them (later, you'll pay attention to the overlap). Jot how many distinct roof / porch styles appear in the first 150 m of view. Rapid change = urban infill zones; homogeneity = single-developer tracts. Pay attention to parallax and the altitude over the roof. Always sanity-check hill distance, not just presence/absence. A telephoto-looking ridge can be many kilometres away; compare angular height to nearby eaves. Slope matters. Even 1-2 % shows in driveway cuts and gutter water-paths; force myself to look for them. Pay relentless attention to camera height and angle. Never confuse a slope and a flat. Slopes are one of your biggest hints - use them! 2 . Clue Categories – reason separately (≤ 2 sentences each) Category Guidance Climate & vegetation Leaf-on vs. leaf-off, grass hue, xeric vs. lush. Geomorphology Relief, drainage style, rock-palette / lithology. Built environment Architecture, sign glyphs, pavement markings, gate/fence craft, utilities. Culture & infrastructure Drive side, plate shapes, guardrail types, farm gear brands. Astronomical / lighting Shadow direction ⇒ hemisphere; measure angle to estimate latitude ± 0.5 Separate ornamental vs. native vegetation Tag every plant you think was planted by people (roses, agapanthus, lawn) and every plant that almost certainly grew on its own (oaks, chaparral shrubs, bunch-grass, tussock). Ask one question: “If the native pieces of landscape behind the fence were lifted out and dropped onto each candidate region, would they look out of place?” Strike any region where the answer is “yes,” or at least down-weight it. °. 3 . First-Round Shortlist – exactly five candidates Produce a table; make sure #1 and #5 are ≥ 160 km apart. | Rank | Region (state / country) | Key clues that support it | Confidence (1-5) | Distance-gap rule ✓/✗ | 3½ . Divergent Search-Keyword Matrix Generic, region-neutral strings converting each physical clue into searchable text. When you are approved to search, you'll run these strings to see if you missed that those clues also pop up in some region that wasn't on your radar. 4 . Choose a Tentative Leader Name the current best guess and one alternative you’re willing to test equally hard. State why the leader edges others. Explicitly spell the disproof criteria (“If I see X, this guess dies”). Look for what should be there and isn't, too: if this is X region, I expect to see Y: is there Y? If not why not? At this point, confirm with the user that you're ready to start the search step, where you look for images to prove or disprove this. You HAVE NOT LOOKED AT ANY IMAGES YET. Do not claim you have. Once the user gives you the go-ahead, check Redfin and Zillow if applicable, state park images, vacation pics, etcetera (compare AND contrast). You can't access Google Maps or satellite imagery due to anti-bot protocols. Do not assert you've looked at any image you have not actually looked at in depth with your OCR abilities. Search region-neutral phrases and see whether the results include any regions you hadn't given full consideration. 5 . Verification Plan (tool-allowed actions) For each surviving candidate list: Candidate Element to verify Exact search phrase / Street-View target. Look at a map. Think about what the map implies. 6 . Lock-in Pin This step is crucial and is where you usually fail. Ask yourself 'wait! did I narrow in prematurely? are there nearby regions with the same cues?' List some possibilities. Actively seek evidence in their favor. You are an LLM, and your first guesses are 'sticky' and excessively convincing to you - be deliberate and intentional here about trying to disprove your initial guess and argue for a neighboring city. Compare these directly to the leading guess - without any favorite in mind. How much of the evidence is compatible with each location? How strong and determinative is the evidence? Then, name the spot - or at least the best guess you have. Provide lat / long or nearest named place. Declare residual uncertainty (km radius). Admit over-confidence bias; widen error bars if all clues are “soft”. Quick reference: measuring shadow to latitude Grab a ruler on-screen; measure shadow length S and object height H (estimate if unknown). Solar elevation θ ≈ arctan(H / S). On date you captured (use cues from the image to guess season), latitude ≈ (90° – θ + solar declination). This should produce a range from the range of possible dates. Keep ± 0.5–1 ° as error; 1° ≈ 111 km.
…and I ran it on a set of increasingly impossible pictures.
Here are my security guarantees: the first picture came from Google Street View; all subsequent pictures were my personal old photos which aren’t available online. All pictures were screenshots of the original, copy-pasted into MSPaint and re-saved in order to clear metadata. Only one of the pictures is from within a thousand miles of my current location, so o3 can’t improve performance by tracing my IP or analyzing my past queries. I flipped all pictures horizontally to make matching to Google Street View data harder.
Here are the five pictures. Before reading on, consider doing the exercise yourself - try to guess where each is from - and make your predictions about how the AI will do.
Last chance to guess on your own . . . okay, here we go.
Picture #1: A Flat, Featureless Plain
I got this one from Google Street View. It took work to find a flat plain this featureless. I finally succeeded a few miles west of Amistad, on the Texas-New Mexico border.
o3 guessed: “Llano Estacado, Texas / New Mexico, USA”.
Llano Estacado, Spanish for “Staked Plains”, is the name of a ~300 x 100 mile region including the correct spot. When asked to be specific, it guessed a point west of Muleshoe, Texas - about 110 miles from the true location.
Here’s o3’s thought process - I won’t post the whole thing every time, but I think one sample will be useful:
This doesn’t satisfy me; it seems to jump to the Llano Estacado too quickly, with insufficient evidence. Is the Texas-NM border really the only featureless plain that doesn’t have red soil or black soil or some other distinctive characteristic?
I asked how it knew the elevation was between 1000 - 1300 m. It said:
So, something about the exact type of grass and the color of the sky, plus there really aren’t that many truly flat featureless plains.
Picture #2: Random Rocks And The Flag Of An Imaginary Country
I was so creeped out by the Llano Estacado guess that I decided to abandon Google Street View and move on to personal photos not available on the Internet.
When I was younger, I liked to hike mountains. The highest I ever got was 18,000 feet, on Kala Pattar, a few miles north of Gorak Shep in Nepal. To commemorate the occasion, I planted the flag of the imaginary country simulation that I participated in at the time (just long enough to take this picture - then I unplanted it).
I chose this picture because it denies o3 the two things that worked for it before - vegetation and sky - in favor of random rocks. And because I thought the flag of a nonexistent country would at least give it pause.
o3 guessed: “Nepal, just north-east of Gorak Shep, ±8 km”
This is exactly right. I swear I screenshot-copy-pasted this so there’s no way it can be in the metadata, and I’ve never given o3 any reason to think I’ve been to Nepal.
Here’s its explanation:
At least it didn’t recognize the flag of my dozen-person mid-2000s imaginary country sim.
Picture #3: My Friend’s Girlfriend’s College Dorm Room
There’s no way it can recognize an indoor scene, right? That would make no sense. Still, at this point we have to check.
This particular dorm room is in Sonoma State University, Rohnert Park, north-central California.
o3’s guess: “A dorm room on a large public university campus in the United States—say, Morrill Tower, Ohio State University, Columbus, Ohio (chosen as a prototypical example rather than a precise claim), […] c. 2000–2007”
Okay, so it can’t figure out the exact location of indoor scenes. That’s a small mercy.
I took this picture around 2005. How did o3 know it was between 2000 and 2007? It gave two pieces of evidence:
“Laptop & clutter point to ~2000-2007 era American campus life”.
“Image quality grainy, low-resolution, colour noise → early 2000s phone/webcam”
Unless college students stopped being messy after 2007, it must be the phone cam.
Picture #4: Some Really Zoomed In Blades Of Grass
Okay, so it’s sub-perfect at indoor scenes. How far can we take its outdoor talent?
This is a zoomed-in piece of lawn from a house I used to rent in Westland, Michigan.
o3’s guess: “Pacific Northwest USA suburban/park lawn.”
Swing and a miss. Its second guess was England, third was Wisconsin. Seems like grass alone isn’t enough.
Picture #5: Basically Just A Brown Rectangle
I figured I’d give it a chance to redeem itself in the “heavily zoomed in outdoor scene” category.
This is a zoomed-in piece of a picture I took of the Mekong River in Chiang Saen, Thailand.
o3’s guess: “Open reach of the Ganges about 5 km upstream of Varanasi ghats. Biggest alternative remains a similarly turbid reach of the lower Mississippi (~15 %), then Huang He or Mekong reaches (~10 % each).”
The Mekong River was its fourth guess for this brown rectangle!
Looking through its chain of thought, it explains why Mekong is only #4:
Lower Mekong has lately swung from brown to an aquamarine cast because upstream dams trap silt. [This doesn’t look] like the near-greyish buff in your image.
This is an old picture from 2008, so that might be what tripped it up. I re-ran the prompt in a different o3 window with the extra information that the picture was from 2008 (I can’t prove that it doesn’t share information across windows, but it didn’t mention this in the chain of thought). Now the Mekong is its #1 pick, although it gets the exact spot wrong - it guesses the Mekong near Phnom Penh, over a thousand miles from Chiang Saen.
Bonus Picture: My Old House
I wondered whether a picture with more information would let it get the exact location, down to street and address.
This is the same picture that furnished the lawn grass earlier - my old house in Westland, Michigan.
o3’s guess: “W 66th St area, Richfield, Minnesota, USA. Confidence: ~40 % within 15 km; ~70 % within the Twin-Cities metro; remainder split between Wisconsin (20 %) and Michigan/Ontario (~10 %).”
Not only couldn’t it get the exact address, but it did worse on this house than on the flat featureless plain!
When I told it about its error, it acted in a very human way - it said that in hindsight, it should have known:
I don’t know what to make of this.
I looked up W 66th Street in Richfield, Minnesota, and it looks so much like my old neighborhood that it’s uncanny.
Yeah, OK, It’s That Good
Kelsey’s experience was neither cheating nor coincidence. The AI really is great.
So is this the thing with the chimp and the helicopter?
After writing this post, I saw a different way of presenting these same results: GeoGuessr master Sam Patterson went head-to-head against o3 and lost. But only by a little. And he let other people try the same image set, and a few (lucky?) people beat o3’s score. So maybe o3 is at the top of the human range, rather than far beyond it, and ordinary people just don’t appreciate how good GeoGuessng can get.
I’m not sure I buy this. First, Kelsey said o3’s performance improved markedly with the special prompt, and Sam didn’t use it. Second, when I tried Sam’s image set, it was much too easy - I (completely untrained) could often get within 10 - 50 miles, and about half of the examples had visible place name signs, including one saying BIENVENIDOS A PUERTO PARRA. This is a recipe for ceiling effects and random meaningless variations in who clicks the exact right sub-sub-sub-spot. I question whether any human could get Kelsey’s beach or my rock pile.
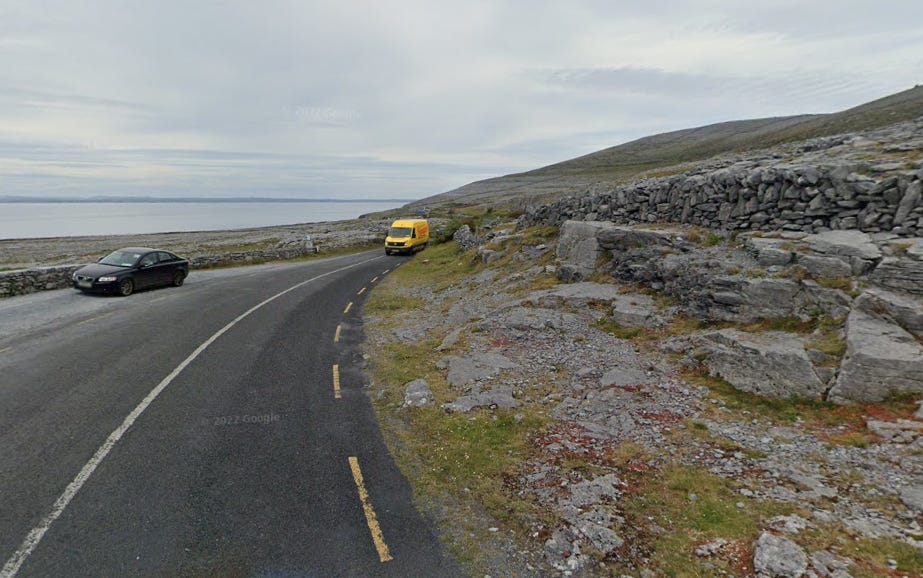
Still, this experience slightly calms my nerves. The AI seems to be using human-comprehensible cues - vegetation, sky color, water color, rock type. It can’t get literally-impossible pictures. It’s just very, very smart.
Is this progress towards a more measured, saner view? Or is it just how things always feel after they’ve happened? Is this the frog-boiling that eventually leads to me dismissing anything, however unbelievable it would have been a few weeks earlier, as “you know, simple pattern-matching”?
If you want to test this for yourself, go to chatgpt.com and register for a free account for access to o3-mini. You may need to pay $20/month to access o3. And if you want to learn more about the differences between OpenAI’s models, and why they have such bad names, see our new post at the AI Futures Project blog.
Share this post